The Impact of Image Annotation Tools in Machine Learning
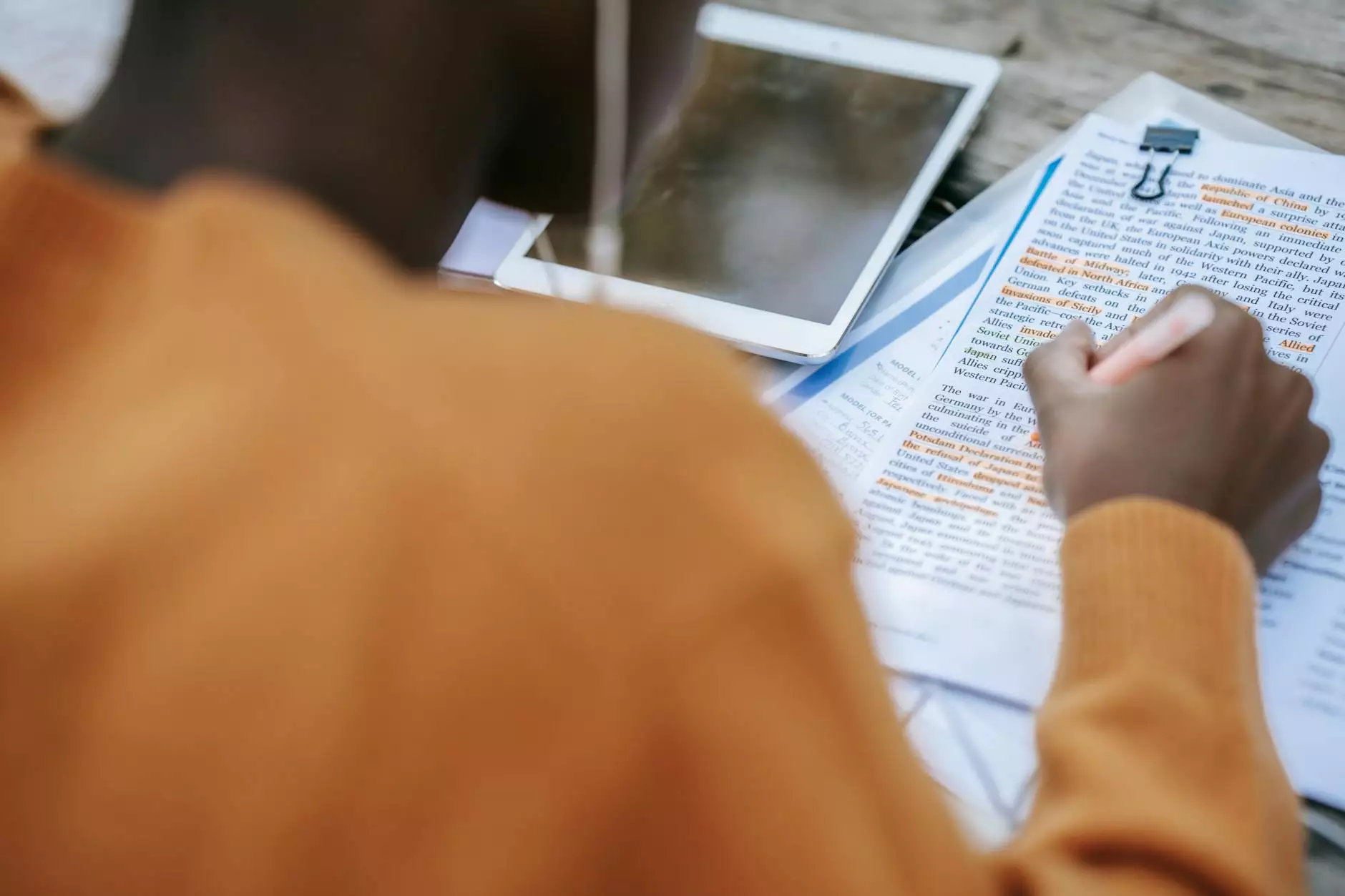
In today's dynamic digital landscape, machine learning is at the forefront of innovation across various industries. One pivotal component that enhances the effectiveness of machine learning algorithms is the image annotation tool. These tools are vital for training machine learning models, especially in fields like computer vision, robotics, and autonomous driving. In this comprehensive guide, we will delve deep into the significance of image annotation tools, their applications, and how they are transforming business operations.
Understanding Image Annotation Tools
Image annotation involves tagging or labeling images to provide context and meaning, which is crucial for enabling machines to comprehend visual data. Image annotation tools streamline this process, allowing businesses to efficiently create annotated datasets that fuel their machine learning models.
Types of Image Annotation
- Bounding Box Annotation: This involves drawing rectangles around objects in images, helping models learn to identify those objects.
- Semantic Segmentation: Here, each pixel of an image is tagged with a class label, providing detailed identification of the object.
- Image Tagging: Adding keywords or tags to an image for easy search and categorization.
- Landmark Annotation: Marking specific points on an image, commonly used in facial recognition.
Importance of Image Annotation in Machine Learning
Effective machine learning relies heavily on the quality and precision of training data. Image annotation plays a critical role in ensuring that models learn accurately. Here’s how:
1. Enhancing Model Accuracy
Annotated images provide the necessary context for machine learning models to recognize and interpret visual data. The image annotation tool machine learning synergy is crucial for developing accurate models. By providing clear, labeled data, these tools mitigate errors that can occur during model training, leading to improved performance.
2. Enabling Computer Vision Applications
From self-driving cars to facial recognition software, computer vision applications require extensive training datasets. Image annotation tools help create large databases of annotated images, enabling advanced algorithms to learn nuances in data, ultimately enhancing application functionality.
3. Streamlining Data Processing
With the rise of automation, image annotation tools simplify the data processing workflow. They allow multiple users to annotate images concurrently, speeding up the timeline for dataset creation. Consequently, businesses can deploy machine learning solutions more rapidly, gaining a competitive edge in their respective fields.
Applications of Image Annotation in Business
Image annotation tools serve various industries, streamlining operations and driving innovation. Let’s explore how different sectors leverage these technologies:
1. Healthcare
In the healthcare sector, image annotation plays a vital role in radiology. Annotated medical images enable algorithms to detect anomalies in X-rays, MRIs, and CT scans. By deploying machine learning tools, healthcare providers enhance diagnostic accuracy, leading to better patient outcomes.
2. Retail and E-commerce
Retail businesses utilize image annotation for several purposes, such as product tagging, inventory management, and improving customer experience. By using annotated images, retailers can develop systems that recommend products based on visual similarities, increasing sales conversion rates.
3. Automotive Industry
As autonomous vehicles become more prevalent, the demand for accurate image annotation tools in the automotive industry has drastically increased. These tools help in training machine learning models that assist vehicles in recognizing road signs, pedestrians, and other vehicles on the road, ensuring safer driving experiences.
4. Security and Surveillance
In security, image annotation tools aid in identifying threats through facial recognition and behavioral analysis. By annotating surveillance footage, security systems powered by machine learning can detect suspicious activities in real time.
Choosing the Right Image Annotation Tool
With numerous options available, businesses must select an image annotation tool that suits their specific needs. Here are some factors to consider:
1. User-Friendliness
The tool should have an intuitive interface that makes it easy for users of all skill levels to annotate images efficiently. Features like drag-and-drop functionality and simple tagging options can significantly improve productivity.
2. Scalability
As your dataset grows, so should your annotation tool's capabilities. Choose a tool that can handle large volumes of images without sacrificing performance.
3. Integration Capabilities
Opt for a tool that integrates seamlessly with existing machine learning platforms and frameworks. This will streamline the workflow and enhance collaboration among teams.
4. Support and Community
Having access to robust customer support and an active user community can be invaluable. It allows users to resolve issues quickly and share best practices.
Future Trends in Image Annotation and Machine Learning
The technology surrounding image annotation is constantly evolving. Here are some trends expected to shape the future of this industry:
1. Automated Annotation Tools
As machine learning advances, we can expect the rise of automated image annotation tools that utilize AI to annotate images with minimal human intervention. These tools will help reduce costs and speed up the annotation process considerably.
2. Increased Use of Synthetic Data
Synthetic data generation, where artificial images are created for use in training, is gaining traction. This method reduces the reliance on real-world data, which can be expensive and time-consuming to annotate. Consequently, businesses can train models without the limitations of traditional datasets.
3. Cross-domain Image Annotation
Cross-domain image annotation is an emerging trend where models trained on one type of data are applied to different domains. This allows businesses to leverage annotations across multiple projects, fostering greater innovation and versatility.
Conclusion
The intersection of image annotation tools and machine learning presents vast opportunities for businesses aiming to innovate and streamline operations. By harnessing the power of accurate annotations, companies can develop cutting-edge solutions that drive efficiency and enhance customer experience. As these technologies continue to evolve, staying ahead of the curve will be paramount for businesses looking to retain their competitive edge in an increasingly automated world.
In summary, the integration of image annotation tools in machine learning is not just a technological trend but a fundamental shift that is reshaping industries. Investing in quality image annotation processes will undoubtedly yield fruitful results, positioning businesses for future success.